Short Introduction
We provide in-depth assessment of the evaluation pipeline for video summaries using two popular benchmark datasets: SumMe [1] and TVSum [2] . Surprisingly, we observe that randomly generated summaries achieve comparable or better performance to the state-of-the-art. In some cases, the random summaries outperform even the reference summaries. Moreover, it turns out that the video segmentation, which is often considered as a fixed pre-processing method, has the most significant impact on the performance measure.
- M. Gygli, H. Grabner, H. Riemenschneider, and L. van Gool, “Creating summaries from user videos,” in European Conference on Computer Vision (ECCV), 2014, pp. 505–520.
- Y. Song, J. Vallmitjana, A. Stent, and A. Jaimes, “TVSum : Summarizing Web Videos Using Titles,” in IEEE Computer Society Conference on Computer Vision and Pattern Recognition (CVPR), 2015, pp. 5179–5187.
Experimental Results
We devise a randomization test to validate evaluation frameworks. In the experiments we generate video summaries that are independent of video content by utilising random importance scores and random video segment boundaries. F1 scores obtained by these randomized (and partially randomized) summaries serve as a baseline that can be achieved completely by chance.
Evaluation on SumMe
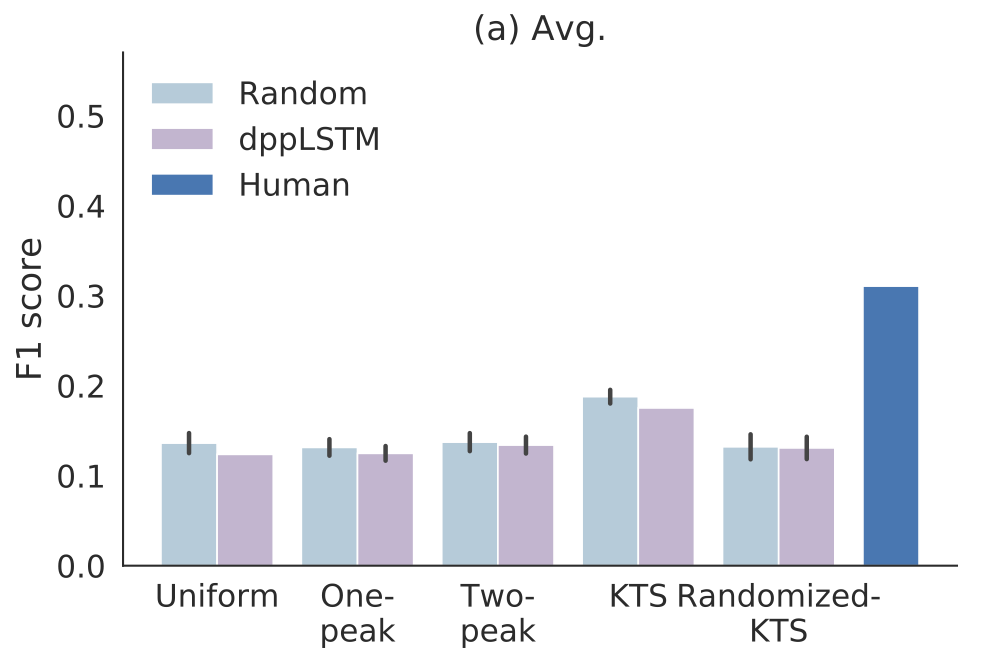
F1 scores for different segmentation and importance score combinations for SumMe. Light blue bars refer to random summaries and dark blue bars indicate scores of manually created reference summaries (leave-one-out test). Purple bars show the scores for DR-DSN importance scoring with different segmentation methods.
Evaluation on TVSum
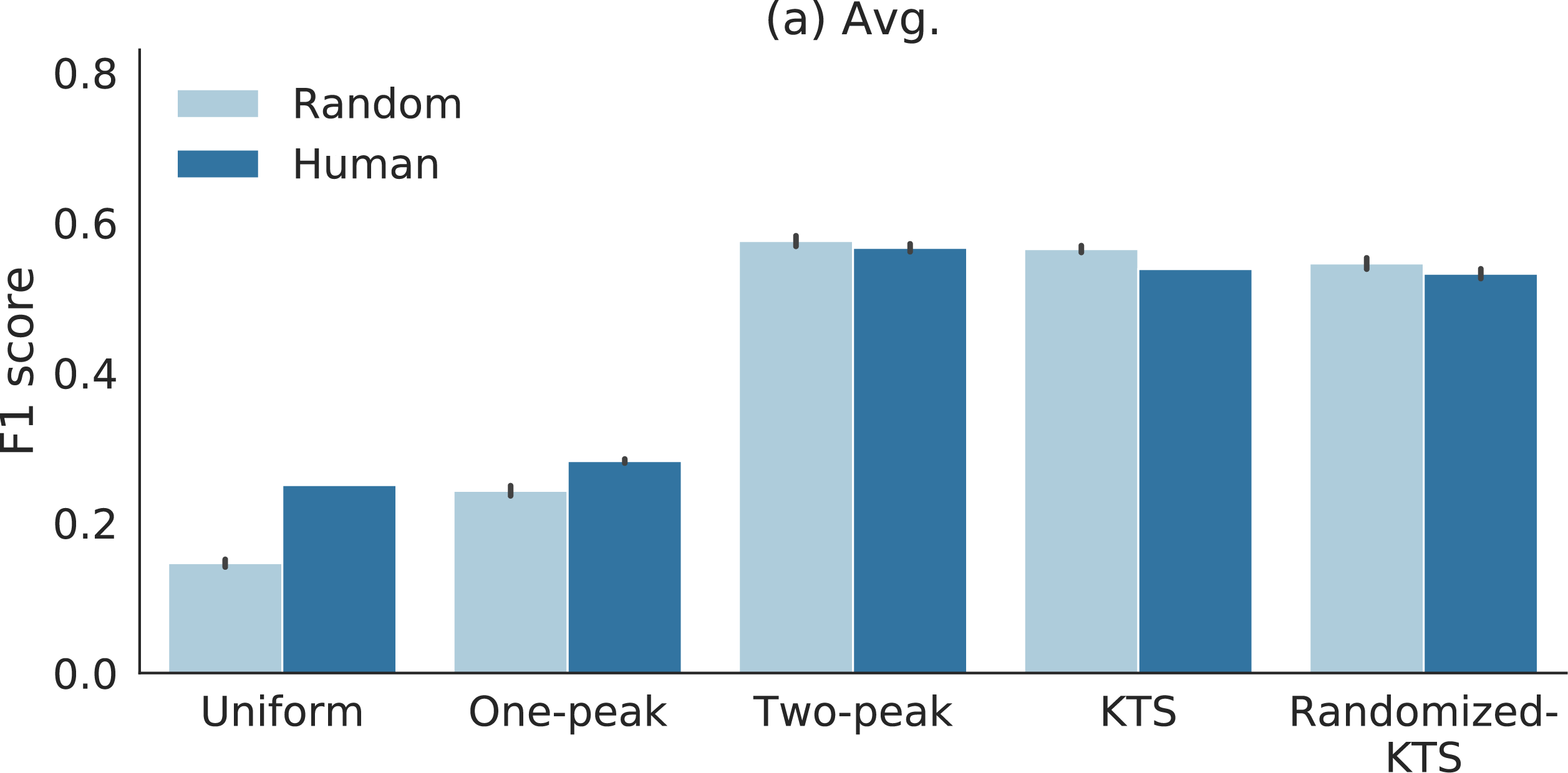
F1 scores for different segmentation methods combined to either random or human annotated importance scores (leave-one-out) for TVSum dataset. Light blue bars refer to random scores and dark blue bars indicates human annotations. Interestingly, the random and human annotations obtain similar F1 scores in most cases.
Effects of Video Segmentation
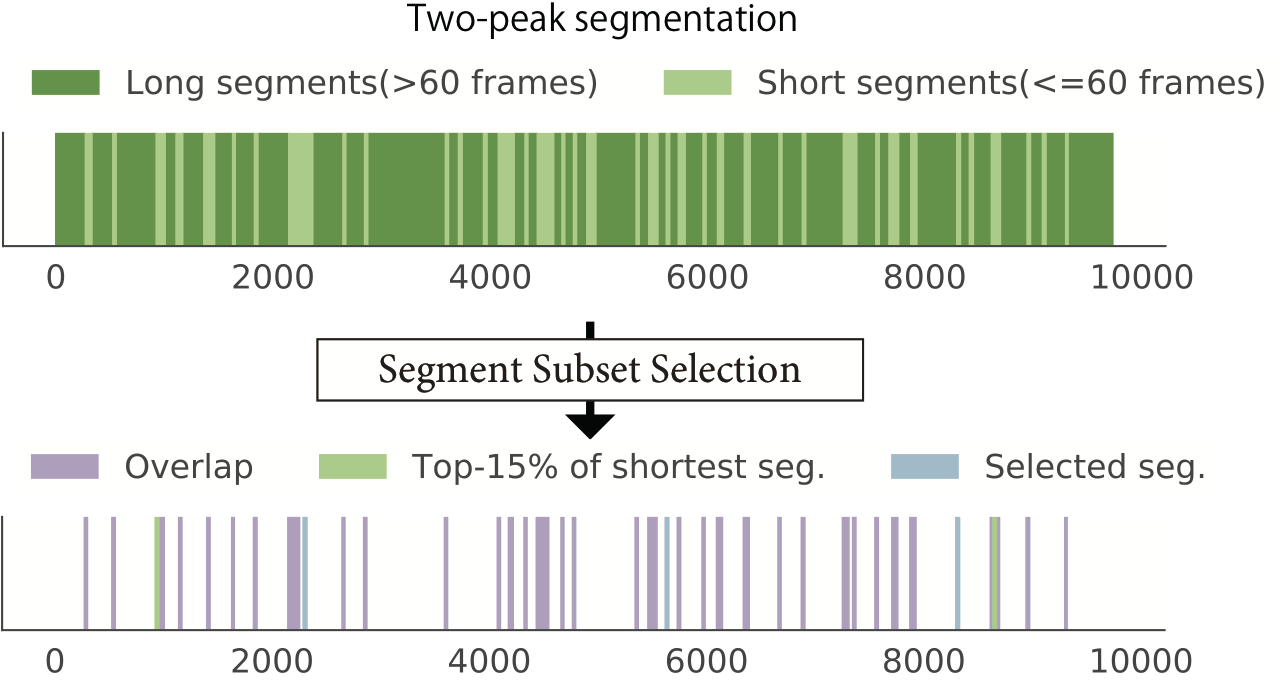
The results can be understood by examining how the segment length affects on selection procedure in the knapsack formulation that is most commonly adopted in video summarization methods. Segment selection as a knapsack problem penalize long segments, thus only short segments are highly likely to be selected.
Paper
Rethinking the Evaluation of Video SummariesCode
mayu-ot/rethinking-evsCitation
@InProceedings{otani2018vsumeval, title={Rethinking the Evaluation of Video Summaries}, author={Mayu Otani, Yuta Nakahima, Esa Rahtu, and Janne Heikkil{\"{a}}}, booktitle={The IEEE Conference on Computer Vision and Pattern Recognition (CVPR)}, year={2019} }